- Speaker
- Prof. Si Wu
- School of Brain and Cognitive Sciences, Beijing Normal University
- Abstract
Owing to its many computationally desirable properties, the model of continuous attractor neural networks (CANNs) has been successfully applied to describe the encoding of simple continuous features in neural systems, such as orientation, moving direction, head direction and spatial location of objects. Recent experimental and computational studies revealed that complex features of external inputs may also be encoded by low-dimensional CANNs embedded in the high-dimensional space of neural population activity. The new experimental data also confirmed the existence of the M-shaped correlation between neuronal responses, which is a correlation structure associated with the unique dynamics of CANNs. This body of evidence suggests that CANNs may serve as a canonical model for neural information representation. In this talk, I will review our researches on CANNs in the past years and look into the future of applying CANNs in brain-inspired computation.
- About the Speaker
吴思, 博士, 教授。1987-1995年在北京师范大学获得广义相对论硕士、统计物理专业博士学位。作为博士后、助理教授、副教授先后工作于香港科技大学、比利时林堡大学、日本理化学所、英国谢菲尔德大学和英国萨斯克斯大学。2008年7月回国,任中囯科学研究院神经科学研究所研究员,入选百人计划。从2011年9月起,任北京师范大学认知神经科学与学习国家重点实验室教授,麦戈文脑科学研究所研究员。研究经历包括:广义相对论、统计物理、人工智能、机器学习和计算神经科学。近十多年的研究主要集中于计算神经科学与机器学习,长期关注于神经系统的信息处理机制,系统发展了记忆的连续吸引子网络模型。已发表论文90余篇,包括Nature Neuroscience,PNAS,PLoS Biology, J.Neurosci.,NIPS等。目前担任Frontiers in Computational Neuroscience主编,Faculty of 1000, Neural Networks, BMC Neuroscience等编委。回国后一直致力于推动计算神经科学在中国的发展,发起组织了一系列相关研讨会和短期课程,如冷泉港-亚洲计算与认知神经科学暑期学校。
- Date&Time
- 2016-01-07 10:00 AM
- Location
- Room: A203 Meeting Room
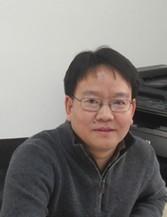