- Speaker
- Prof. Tie-Jun Li
- School of Mathematical Sciences, Peking University
- Abstract
Detecting direct dependencies/associations between variables in a network based on the observed data is of great importance in the studies of various biological networks and relationships. Through multiscale association analysis, we show why conditional mutual information (CMI)/partial correlation (PC) suffers from an underestimation problem for networks with strong correlations, and we further resolve this issue with a new measure, partial association (PA), derived from multiscale conditional mutual information. Linear and nonlinear versions of PA correspond to PC and CMI respectively, and they are shown to be able to accurately quantify direct dependencies or construct biological networks from both theoretical and computational viewpoints. The effectiveness of our method is also validated by survival and functional analyses on TCGA data.
- About the Speaker
李铁军, 北京大学数学科学学院教授, 教育部新世纪人才支持计划及国家自然科学基金委优秀青年基金获得者。研究领域为随机模型、理论及算法, 近年来主要关注于化学反应随机动力学的稀有事件, 发展了化学反应随机系统的两尺度大偏差及生物体系能量景观理论, 在复杂网络模型约化及复杂流体的随机模型分析等方面也取得了重要成果。
- Date&Time
- 2017-11-23 3:00 PM
- Location
- Room:A203 Meeting Room
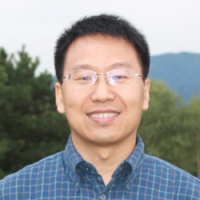