- Speaker
- Prof. Jian-Lin Xia
- Purdue University
- Abstract
It has been known in potential theory that, for some kernels matrices corresponding to well-separated point sets, fast analytical low-rank approximation can be achieved via the use of proxy points. This proxy point method gives a surprisingly convenient way of explicitly writing out approximate basis matrices for a kernel matrix. However, this elegant strategy is rarely known or used in the numerical linear algebra community. It still needs clear algebraic understanding of the theoretical background. Moreover, rigorous quantifications of the approximation errors and reliable criteria for the selection of the proxy points are still missing. In this work, we use contour integration to clearly justify the idea in terms of a class of important kernels. We further provide comprehensive accuracy analysis for the analytical compression and show how to choose nearly optimal proxy points. The analytical compression is then combined with fast rank-revealing factorizations to get compact low-rank approximations and also to select certain representative points. We provide the error bounds for the resulting overall low-rank approximation. This work thus gives a fast and reliable strategy for compressing those kernel matrices. Furthermore, it provides an intuitive way of understanding the proxy point method and bridges the gap between this useful analytical strategy and practical low-rank approximations. This is joint work with Xin Ye and Lexing Ying.
- About the Speaker
Prof.Xia' homepage: http://www.math.purdue.edu/~xiaj/
- Date&Time
- 2019-12-24 9:00 AM
- Location
- Room: A203 Meeting Room
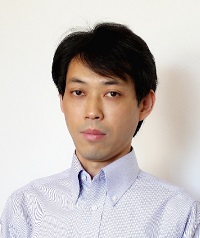