- Speaker
- A/Prof. Si-Ye Li
- 军事科学院军事智能研究院
- Abstract
With powerful processing and representation capabilities for arbitrary data structures, data-driven-based graph neural networks (GNNs) have received increasing and significant interest in solving various mechanical problems. However, the application of DNNs/GNNs for flow simulations has mainly concentrated on relatively simple cases of incompressible flows. The strongly discontinuous structures that appear in compressible flows dominated by convection, such as shock waves, introduce significant challenges when approximating the nonlinear solutions or governing equations. This talk will present a novel flow-field-message-informed graph neural network (FFMI-GNN) for spatio-temporal simulations of compressible flows. Based on the "mechanics knowledge + data driven" perspective, the inherent consistency between CFD methods and GNN methods are revealed. To enhance the model's ability to learn and predict strong discontinuous solutions, the shock detector method embedded into the graph representations is employed to extract the local flow field nonlinear message, which is fused with the physical and spatial messages of flows. Moreover, a transfer-learning- based high-resolution flow field reconstruction method with multi-heads graph attention (TL-MHGAT) is proposed for multi-scale flow fields. By exploiting a multi-head graph attention mechanism and a multi-head perceptron decoding strategy, the messages with different scales are effectively captured and the mutual influence of physical quantities of different feature scales is avoided. Extensive numerical tests have been conducted in to validate the effectiveness and generalizability of the proposed GNN-based methods.
- About the Speaker
李思冶博士, 军事科学院军事智能研究院(AMII)助理研究员, 分别于2019和2024年在火箭军工程大学获得工学学士和博士学位。主要研究方向为可压缩流高精度数值方法和智能流体求解方法, 发展了色散与耗散特性独立自适应的高精度有限差分方法, 并扩展于三维高分辨率有限体积格式, 用于高性能武器装备的空气动力特性研究。提出了力学知识信息嵌入的图神经网络力学求解框架, 并用于可压缩流动、超音速机翼、无人机控制与微分博弈等项目研究中。已发表10 余篇论文, 包括在JCP, POF, JSC, Computer & Fluids上发表7篇。曾获第二十届全国计算流体力学大会优秀青年论文一等奖等荣誉。
- Date&Time
- 2025-05-19 2:00 PM
- Location
- Room: A203 Meeting Room
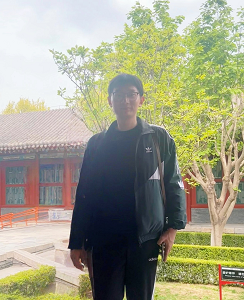